The Marshall-Olkin Kappa distribution: Properties and applications
⁎Corresponding author. maria_mavi786@yahoo.com (Maria Javed)
-
Received: ,
Accepted: ,
This article was originally published by Elsevier and was migrated to Scientific Scholar after the change of Publisher.
Peer review under responsibility of King Saud University.
Abstract
This study proposes a new competitive model, the Marshall-Olkin Kappa distribution and presents its various properties. These properties include the derivation of probability density function, quantiles, rth moment, mode, mean deviation and survival function. Results for the various inequality indices are obtained. Expression regarding order statistics is given. The unknown parameters of the proposed distribution are estimated using the maximum likelihood estimation method. Empirical illustration is provided using two real life data sets.
Keywords
Kappa distribution
Marshall-Olkin distribution
Moments
Reliability
Entropy
Estimation
1 Introduction
Kappa distribution is a good choice for model fitting among its competitors in presence of extreme values. The study of extreme events like floods, cyclone and heavy rains is necessary in the planning of water relevant setups, cultivation of crops in agriculture, climatic conditions, overseeing environmental changes and floods basic control systems.
Mielke (1973) and Mielke and Johnson (1973) introduced a class of asymmetric positively skewed distribution, used for explaining and examining rainfall data and weather modifications which obtained attention from the hydrologist. This class of distribution was named as the three parameter kappa distribution. Conventionally, the log normal and the gamma distributions are fitted to precipitation data but these distributions have their own limitations due to non-existence of closed forms of cdfs and quantile functions. Closed algebraic expressions can be analyzed with the help of the class of kappa distribution.A random variable
The corresponding probability density function is
Hussain (2015) proposed three extended forms of kappa distribution namely Exponentiated generalized kappa, Kumaraswamy generalized kappa and McDonald generalized kappa distribution. He explored different statistical properties, survival properties, inequality indices and entropies of new extended forms. He used different methods to estimate the unknown parameters of new forms. He also checked the efficiency of the proposed models of kappa distribution with some real life data sets.
The study in hands is extended as follows. In Section 2, we give a brief introduction of parameter induction in probability distributions and one of the generated family of distribution i.e. Marshall-Olkin (MO) distribution. In Section 3, we introduced a new generalization of the kappa distribution, namely, the Marshall-Olkin Kappa (MOK) distribution. We derived the statistical properties like quantile function, median, mode, moments, moment generating function (mgf), characteristic function (cf), mean deviation from mean and from median and reliability properties i.e. survival function, hazard rate function (hrf), reversed hazard rate and mean residual life function of MOK distribution. We give expressions of inequality measures including Lorenz curve, Bonferroni curve, Zenga index, Atkinson index, Pietra index and generalized entropy. Most famous and useful method i.e. method of maximum likelihood is used to estimate the unknown parameters of MOK distribution. In Section 4, proposed MOK distribution is applied on two real data sets. Different model selection criterions are used to compare proposed and existing distributions on the same data sets. This compatibility is also shown with the help of graphical representation.
2 Parameter induction in continuous univariate distribution
There is an accelerating trend of inducting shape parameter(s) to the basic distribution in advancing generated families. Doubtlessly the popularity and the utilization of incomplete functions in generated distributions have attracted the thought of statisticians, mathematicians, researchers, engineers, financial specialists, demographers and other connected scientists. Diverse explanations for this thought may be
-
The computational and diagnostic facilities available through programming softwares like R (packages), ox5, Python, Matlab, Maple and Mathematica. With the assistance of these softwares scientists can easily deal with the issues in calculating incomplete functions.
-
To analyze the tail properties of distributions more extensively one can introduce additional shape parameters to the underlying distribution.
-
The goodness of fit can be enhanced with addition of shape parameters.
-
Distributions with additional shape parameters performs better in case of skewed data as compared to the conventional distributions (Pescim et al. 2012).
2.1 General theory of Marshall-Olkin distribution
Marshall and Olkin (1997) presented an elastic semi-parametric family of distributions. They defined a new survival function
The survival function
The associated cdf and pdf of MO distribution are
Marshall-Olkin generalizations of different distributions exist in the literature. Some of them are mentioned in our manuscript. Cordeiro and Lemonte (2013) studied the mathematical properties and applications of the Marshall-Olkin extended (MOE) weibull distribution. Other examples include MOE pareto distribution (Alice and Jose, 2003), MOE gamma distribution (Ristić et al., 2007), MOE lomax distribution (Ghitany et al., 2007a), MOE weibull distribution and its application to censored data (Ghitany et al., 2007b), MOE normal distribution (Garcia et al., 2010), MOE lindley distribution (Ghitany et al., 2012), MOE fréchet distribution (Krishna et al., 2013), and MOE birnbaum–saunders distribution (Lemonte, 2013). General properties of the MOE family of distributions were studied recently by Barreto-Souza et al. (2013) and Cordeiro et al. (2014).
3 Marshall-Olkin Kappa (MOK) distribution
Consider the cdf and pdf of kappa distribution from (1.1) and (1.2) then applying the (2.1) and (2.2), we have the Marshall-Olkin Kappa distribution with cdf and pdf given in (3.1) and (3.2)
3.1 Sub models of MOK distribution
-
In case
in (3.2), the MOK distribution reduces to KAP-III distribution. -
If
in (3.2), the MOK distribution reduces to KAP-II distribution.
Graphical representation of pdf and cdf for various values of parameters chosen arbitrary are provided in Figs. 3.1 and 3.2 respectively.
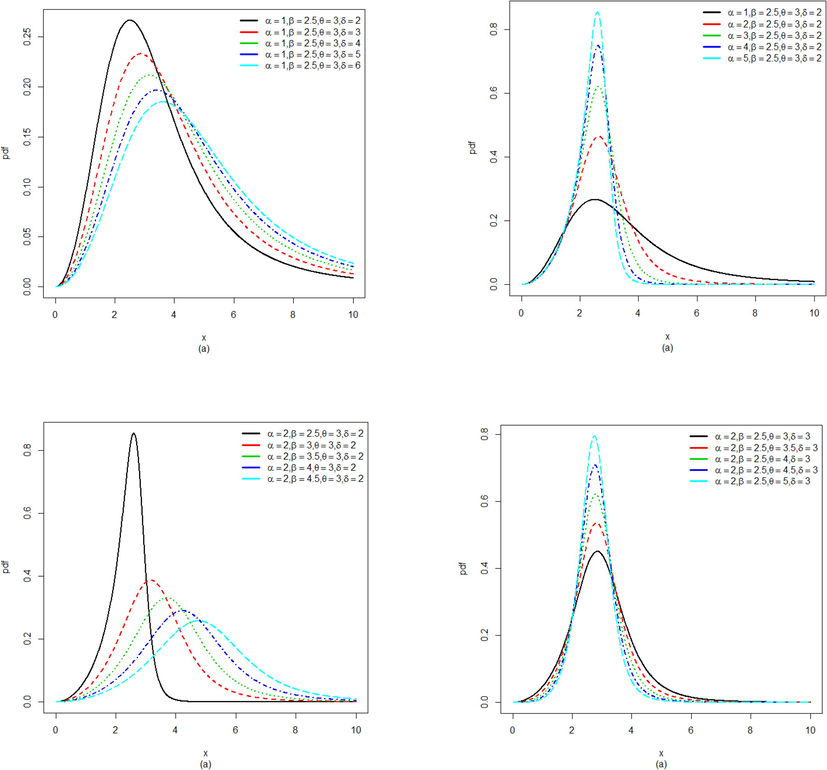
- Graphs of the pdf of MOK distribution.
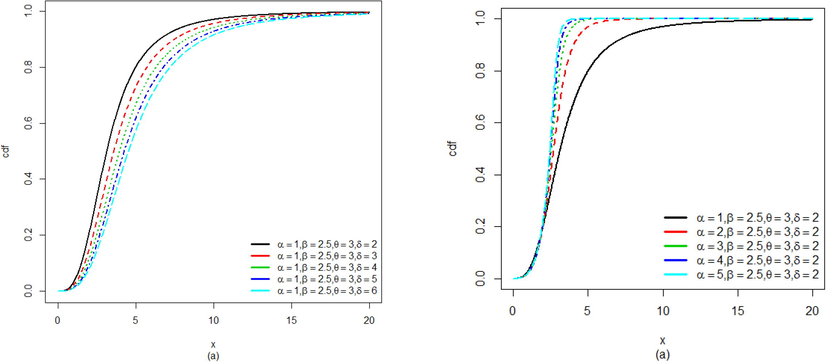
- Graphs of the cdf of MOK distribution.
Some important findings from graphs in Fig. 3.1 are
-
The density function of MOK distribution tends to normal distribution as the value of
increases, while the values of are kept constant. Similar pattern is seen with increase in value of for fixed values of . -
being the shape parameter changes the shape of density function for fixed values of . Increase in makes the density function more leptokurtic. Similar behavior is found for increase in , by fixing the values of .
From Fig. 3.2, graphs of the cdf of MOK distribution satisfy the following properties.
-
goes to 0 as gets smaller -
Conversely
-
is non-decreasing.
The mode of the MOK distribution is the solution of the equation
Taking first derivative of the logarithm of (3.2), we have
Equating to zero and simplifying, we get
It is important to mention that for
3.2 Quantiles and moments
The qth quantile of the MOK distribution is
The median, first and third quartiles of MOK distribution are
The
Using beta function,
MOK distribution has the following moment generating function and characteristic function
3.3 Mean deviation
The amount of variability in a distribution can be measured up to some extent with the help of totality of deviations about the mean and about the median.
If
Substituting
The Mean deviation about mean and Mean deviation about median can be obtained by
Hence, we get
3.4 Reliability properties
Let
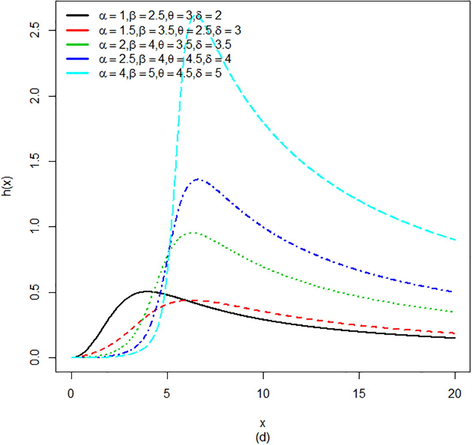
- Graph of the hrf of MOK distribution.
The survival function S(t), cumulative hazard rate function
3.5 Mean residual life function
In many fields like biomedical science, insurance and industrial reliability, mean residual life function is an imperative consideration. It can be obtained through the expression below
Mean residual life function of MOK distribution is given as
3.6 Inequality measures
Inequality measures play key role in many fields like economics to study income and poverty, demography, insurance and medicine. Some are discussed in this study.
3.6.1 An American economist Lorenz (1905) developed a graphical diagram of wealth distribution called Lorenz curve. Lorenz index is defined as
The Lorenz curve is the plot of Lorenz index L(p) verses x, given below is the Lorenz index for MOK distribution.
On the diagram perfect equality of wealth distribution is depicted by a straight diagonal line and a line lies under it shows the true wealth distribution. The difference between two above stated lines is actually the inequality of wealth distribution.
3.6.2 A measure of income inequality was projected by Bonferroni (1930), founded on partial means, that is needed when the main source of income inequality is the occurrence of units whose income is much beneath those of others. The Bonferroni index can be determined through the relation
The Bonferroni curve is the plot of Bonferroni index BC(p) verses
3.6.3 The Zenga index denoted by
3.6.4 Atkinson (1970) proposed an index ranges from 0 to 1 where 0 means an equal wealth distribution. Mathematically Atkinson index can be defined as
Atkinson index for MOK distribution is given by
3.6.5 Pietra (1915) offered an index, known as Schutz index or half of the relative mean deviation. Pietra index is defined as
Expression for the Pietra index
3.7 Generalized entropy (GE)
Variation of the uncertainty can be measured for a random variable through entropy. The generalized entropy (GE) index, suggested by Cowell (1980) and Shorrocks (1980) is
The GE for MOK distribution can be obtained as below
3.8 Order statistics
In many areas of statistical theory and applications, order statistics have significant role. Consider
Inserting pdf and cdf of Kappa distribution and simplifying we get
3.9 Estimation of parameters
Section given below considers the famous and most useful method for estimating the parameters
Partially differentiating
We have four equations as
4 Application of the MOK distribution
A comparison of proposed MOK distribution has been made with the Exponentiated generalized kappa distribution, Kumaraswamy generalized kappa distribution, McDonald generalized kappa distribution, two parameters kappa and three parameters kappa distribution with the help of data sets given in Sections 4.1 and 4.2.
Goodness of fit is generally decided utilizing a likelihood ratio approach. We used two goodness of fit criterions Cramer-Von Mises
4.1 On stream flow amounts (1000 acre-feet)
Following data set (Mielke and Johnson, 1973) consists of stream flow amounts (1,000 acre-feet) for 35 years (1936–70) at the U.S. Geological Survey (USGS) gaging station number 9–3425 for 1st April to 31st August of each year.
192.48, 303.91, 301.26, 135.87, 126.52, 474.25, 297.17, 196.47, 327.64, 261.34, 96.26, 160.52, 314.60, 346.30, 154.44, 111.16, 389.92, 157.93, 126.46, 128.58, 155.62, 400.93, 248.57, 91.27, 238.71, 140.76, 228.28, 104.75, 125.29, 366.22, 192.01, 149.74, 224.58, 242.19, 151.25.
4.2 On failure times of mechanical components
Following data represents the failure times of mechanical components obtained from Silva et al. (2015).
0.040, 1.866, 2.385, 3.443, 0.301, 1.876, 2.481, 3.467, 0.309, 1.899, 2.610, 3.478, 0.557, 1.911, 2.625, 3.578, 0.943, 1.912, 2.632, 3.595, 1.070, 1.914, 2.646, 3.699, 1.124, 1.981, 2.661, 3.779, 1.248, 2.010, 2.688, 3.924, 1.281, 2.038, 2.823, 4.035, 1.281, 2.085, 2.890, 4.121, 1.303, 2.089, 2.902, 4.167, 1.432, 2.097, 2.934, 4.240, 1.480, 2.135, 2.962, 4.255, 1.505, 2.154, 2.964, 4.278, 1.506, 2.190, 3.000, 4.305, 1.568, 2.194, 3.103, 4.376, 1.615, 2.223, 3.114, 4.449, 1.619, 2.224, 3.117, 4.485, 1.652, 2.229, 3.166, 4.570, 1.652, 2.300, 3.344, 4.602, 1.757, 2.324, 3.376, 4.663
Tables 4.1 and 4.2 present the maximum likelihood estimates of the parameters of the distributions and their standard errors in parenthesis. Table 4.3 shows the minimum values of the log likelihood function
Distribution | α | β | θ | δ | a | b |
---|---|---|---|---|---|---|
K2 | 10.7420 (5.9393) |
312.1797 (46.5958) |
– | – | – | – |
K3 | 0.0457 (0.2369) |
161.5442 (15.9801) |
56.7357 (287.0343) |
– | – | – |
McGK | 0.1951 (0.5998) |
24.1709 (53.9753) |
4.3609 (13.2095) |
0.0024 (23.2242) |
42.7565 (99.1328) |
7.5042 (20.1226) |
KGK | 0.1678 (1.0243) |
17.3661 (219.7929) |
7.2191 (44.7869) |
– | 37.6739 (563.6182) |
4.8300 (9.7572) |
EGK | 0.0264 (0.0118) |
42.6137 (39.2628) |
34.2247 (145.7587) |
– | 3.2326 (19.3128) |
79.3117 (102.2513) |
MOK | 0.0626 (0.1406) |
129.8663 (34.1181) |
50.1215 (111.7535) |
2.9310 (3.7595) |
– | – |
(Standard errors in parenthesis).
Distribution | α | β | θ | δ | a | b |
---|---|---|---|---|---|---|
K2 | 17868.2697 (13831.0322) |
4.66187 (0.0015) |
– | – | – | – |
K3 | 17060.1568 (4510.9833) |
4.6624 (0.0012) |
1.3066 (0.1427) |
– | – | – |
McGK | 29.1913 (176.2242) |
4.6808 (0.0823) |
34.1854 (78.5917) |
0.0274 (0.1189) |
0.0473 (0.1089) |
1.3184 (0.2988) |
KGK | 23.9728 (125.6004) |
4.6815 (0.0806) |
34.7390 (112.8132) |
– | 0.0453 (0.1473) |
1.3333 (0.3046) |
EGK | 6.0757 (1.0824) |
5.9738 (0.3541) |
4.9868 (0.7825) |
– | 11.5468 (2.8778) |
0.3326 (0.0788) |
MOK | 6.0909 (5.4648) |
1.4164 (0.7806) |
0.7576 (0.7299) |
15.4506 (30.1753) |
– | – |
(Standard errors in parenthesis).
Data | Stream flow amounts | Failure times of mechanical components | ||||
---|---|---|---|---|---|---|
Distribution |
|
|
|
|
|
|
K2 | 214.1449 | 1.0876 | 0.1802 | 129.3613 | 1.4302 | 0.2574 |
K3 | 207.0037 | 0.4919 | 0.0799 | 126.2338 | 1.3418 | 0.2397 |
McGK | 206.3862 | 1.4356 | 0.2222 | 125.2638 | 1.5101 | 0.1786 |
KGK | 206.4671 | 0.4555 | 0.0788 | 125.2886 | 1.0720 | 0.1864 |
EGK | 206.8389 | 0.4761 | 0.0782 | 127.3661 | 0.7816 | 0.1191 |
MOK | 206.6291 | 0.4583 | 0.0774 | 129.8588 | 0.6006 | 0.0802 |
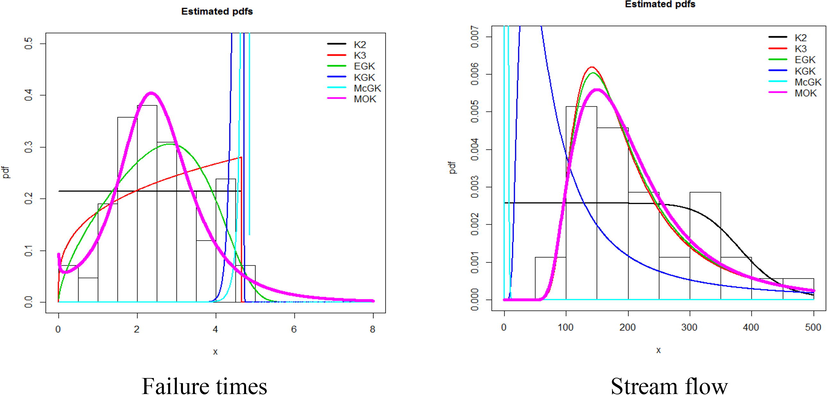
- Graphs of the estimated pdfs for G-Kappa distributions.
5 Concluding remarks
In this manuscript, we proposed a new generalization of kappa distribution named as Marshall-Olkin Kappa (MOK) distribution. Statistical properties i.e. pdf, cdf, mode, median, quantiles, moments and mean deviations of the new model are derived. Various reliability properties, inequality measures and generalized entropy are also studied. Maximum likelihood method is applied to estimate the unknown parameters of the new model. Two real life data sets are used to show the competitiveness of proposed model and finally conclusion has been made that new model may serve better than other competing models.
References
- General results for the Marshall and Olkin’s family of distributions. Ann. Braz. Acad. Sci.. 2013;85:3-21.
- [Google Scholar]
- Elementi di statistica generale. Firenze: Libreria Seber; 1930.
- The Marshall-Olkin family of distributions: mathematical properties and new models. J. Stat. Theor. Pract.. 2014;8:343-366.
- [Google Scholar]
- On the structure of additive inequality measures. Rev. Econ. Stud.. 1980;47:521-531.
- [Google Scholar]
- A new skew generalization of the normal distribution: properties and applications. Comput. Stat. Data. An.. 2010;54:2021-2034.
- [Google Scholar]
- Marshall-Olkin extended Lomax distribution and its application to censored data. Commun. Stat-Theor. M.. 2007;36:1855-1866.
- [Google Scholar]
- Marshall-Olkin extended Weibull distribution and its application to censored data. J. Appl. Stat.. 2007;32:1025-1036.
- [Google Scholar]
- Marshall-Olkin extended Lindley distribution and its application. Int. J. Ap. Mat.. 2012;25:709-721.
- [Google Scholar]
- Hussain, S., 2015. Extension, Properties and Application of Kappa Distribution. M.Phil. Thesis, Submitted to Government College University, Faisalabad, Pakistan. (Unpublished).
- The Marshall-Olkin Fréchet distribution. Commun. Stat-Theor. M.. 2013;42:4091-4107.
- [Google Scholar]
- A new extension of the Birnbaum-Saunders distribution. Braz. J. Probab. Stat.. 2013;27:133-149.
- [Google Scholar]
- Method of measuring the concentration of wealth. J. Am. Stat. Assoc.. 1905;9:209-219.
- [Google Scholar]
- A new method for adding a parameter to a family of distributions with application to the exponential and Weibull families. Biometrika.. 1997;84:641-652.
- [Google Scholar]
- Another family of distributions for describing and analyzing precipitation data. J. Appl. Meteorol.. 1973;12(2):275-280.
- [Google Scholar]
- Three parameter kappa distribution maximum likelihood estimations and likelihood ratio tests. Mon. Weather Rev.. 1973;101:701-707.
- [Google Scholar]
- Stochastic orders of the Marshall-Olkin extended distribution. Stat. Probabil. Lett.. 2012;82:295-302.
- [Google Scholar]
- The new class of Kummer beta generalized distributions. SORT-Stat. Oper. Res. T.. 2012;36:153-180.
- [Google Scholar]
- Delle relazioni fra indici di variabilita, note I e II. Atti del Reale Istituto Veneto di scienze. Lettere ed Arti.. 1915;74:775-804.
- [Google Scholar]
- A Marshall-Olkin gamma distribution and minification process. STARS: Stress and Anxiety Research. Society.. 2007;11:107-117.
- [Google Scholar]
- A new extended gamma generalized model. Int. J. Pure Appl. Math... 2015;100(2):309-335.
- [Google Scholar]
- The Class of additively decomposable inequality measures. Econometrica.. 1980;48:613-625.
- [Google Scholar]
- Proposta per un indice di concentrazione basto sui rapporti fra quantili di populizone e quantili ti reddito. Giornale degli economisti e Annali di Economia. 1984;48:301-326.
- [Google Scholar]