Translate this page into:
Nonlinear optimization in bi-level selective maintenance allocation problem
⁎Corresponding author. irfii.st@amu.ac.in (Irfan Ali)
-
Received: ,
Accepted: ,
This article was originally published by Elsevier and was migrated to Scientific Scholar after the change of Publisher.
Peer review under responsibility of King Saud University.
Abstract
The structural diversity of systems and the resource limitations, and the need to improve system reliability give birth to several optimization techniques and analyses. Recently, the significance of reliability and maintainability concepts has been increasing attention. Several optimization concepts exist in the reliability literature. However, less concern has been given to the bi-level programming concept, especially in the selective maintenance allocation problem. Hence, the present study is motivated and focuses on bridging the existing literature gap. This paper aims to transform a problem of reliability into a Bi-Level Programming Problem (BLPP). The objective functions are non-linear, and the constraints are linear. The current paper demonstrates the reliability optimization problem as a BLPP and applies the Kuhn-Tucker approach to solving the formulated BLPP. A section for estimating the reliability parameters are discussed, and a numerical example has been provided to illustrate the solution procedure.
Keywords
Bi-level programming problem
Selective maintenance allocation problem
Reliability optimization
K-T Conditions
Nonlinear optimization
1 Introduction
Nowadays, engineers design highly complex systems to function reasonably without failure for some time and meet their functional requirements. The absence of complete failure of the system components during its mission cannot be guaranteed. However, its reliability can be measured and ascertain based on the operational lifetime. The reliability of a system component is its failure-free probability during the operational face up to a specific period under certain conditions. Recently, this area is receiving attention from several researchers because of its importance in all real-life operational systems such as manufacturing, transportation, power, telecommunication, and exploration Rausand (2014) and Muhuri et al. (2019).
Several systems have different configurations, and maximizing their overall performance requires Reliability Optimization (RO). The RO problems are of three categories (i) “redundancy allocation problem (RAP)”, (ii) “reliability allocation problem”, and (iii) “Reliability-Redundancy Allocation Problem (RRAP)” Modibbo et al. (2021).The RO has a different view regarding the system structure, which could be series, parallel, series–parallel, k-out-of n system, etc. The Reliability Block Diagram (RBD) of some system structures is shown in Fig. 1.Some systems structural configuration.
The importance of reliability and maintenance theory is significantly increasing in recent times. Engineers, scientists, researchers and industrial managers realized the importance of this topic now more than anytime in history, especially with the advent of advanced technology and soft-computing power. A reliable system optimizes cost and guarantees a design for high-quality products. As a result, it optimizes profit, increases product durability and market acceptability.
Most of the researches in RO concentrate on single optimization of the system reliability; however, there is no significant attention to Bi-level Optimization (BO) of the problem, and the problem can perfectly fit bi-level optimization. Motivated by this fact, this study seeks to present a BO in addressing the selective maintenance allocation problem in RO using bi-level programming. There are many real-life situations where decision-making takes place at levels. In BO, it involves only two levels-the upper and lower. Each level may have a different objective function with decision space in part of the other levels.
Additionally, one level‘s decision may influence the decisions at the other levels; as a result, the objective function of the other level improves. For instance, allocating resources or selective maintenance of some lower components of a system may directly confer benefits on the other levels of the system. The common feature of these systems is that they have an interactive decision-making unit within their structure hierarchically. Each lower-level subsystem executes its function after and following the upper-level subsystem actions. Each subsystem optimizes its reliability independently with other subsystems but may be affected by the performance of those subsystems. The decision-makers problem‘s external effect reflects both the objective function and the feasible solution sets. These processes and procedures are well-explained in Section 3.1. The related literature is reviewed in the following section, and the research gap is established.
1.1 Paper organization
This article’s organization is as follows: In Section 1, the introduction, background and motivation of this research are presented for easy understanding. Section 2 reviewed the related literature and established the gap necessitating this research. Section 3 presents the methodology of the paper discussing the bi-level programming, the K-T optimality conditions and the mathematical formulations. Section 4 discusses the concept of the bi-level selective maintenance allocation problem in reliability optimization and presents its mathematical formulation. The concept is illustrated with a numerical example in Section 5. The results of the illustrations are discussed, and the article is concluded in Section 6 suggesting managerial implications and further scope for investigation.
2 Literature review and research gap
Several studies use the concept of bi-level programming in different sectors. For instance, Sadati et al. (2013) uses BLPP to optimize the random portfolio under a fuzzy environment based on possibility and necessity models. They used newly generated stock market information to calculate the objective function’s upper bound (level) while the lower level is calculated based on historical data. Kornai et al. (1965) applied the concept as a two-level planning problem in game-theoritical model involving two players’ team. Similarly, Bialas et al. (1984) formulated BLPP to decentralized planning as a decision-making process with geometric characteristics and algorithms. Nath et al. (2017), Muhuri et al. (2019) and Sinha et al. (2014) studied a reliability redundancy allocation problem of a series–parallel system and formates a mixed-integer non-linear model based on the bi-level programming concept-the solution to the optimization problem obtained with the help of an evolutionary algorithm using quadratic approximations. In the study, they considered two objective functions as reliability weight and cost.
Nath et al. (2019) conducted similar research to study the effect of “BrainStorm Optimization Algorithm (BSOA) and ”Brainstorm Optimization algorithm in Objective Space (BSOA-OS)”. The study compares these algorithms with ”genetic algorithm (GA) and self-organizing migrating algorithm (SOMA)” and concluded that BSO-OS converges faster. Most recently, Ghasemi et al. (2021) formulated a bi-level mathematical model in response to the COVID-19 pandemic for logistic management considering the evolutionary game with environmental feedback. Similarly, Li et al. (2021) proposed a stochastic bi-level model for the facility location and protection problem having a probabilistic interdiction strategy. Candler et al. (1982) formulated a Stackelberg game as a linear two-level programming problem where the first player optimizes his objective function. However, the constraints are under the control of the second player. The study uses a necessary condition to show that a local optimum exists in the game. Sakawa et al. (2012) extended the Stackelberg solution to consider decision-makers fuzzy goals in a randomized fashion and solve the problem via maximizing the probability with possibility.Gupta et al. (2018) also applied a bi-level concept in studying supply chain network order allocation problem under fuzziness. The authors formulated the problem as multi-objective bi-level optimization. Fortuny-Amat et al. (1981) presented an economic interpretation of a two-level programming problem in which the Khun-Tucker conditions replace the lower level problem and transformed using mixed-integer quadratic programming problem exploring and demonstrating the complementary slackness conditions. Sakawa et al. (2002) formulated decentralized BLPP using an interactive fuzzy programming approach. Similarly, Wen et al. (1990) formulated BLPP in which the yes or no high-level decision-makers control combined with the real-valued lower-level decision control as a mixed-integer programming problem. The study provides both exact and heuristics algorithms for solving such models.
Christiansen et al. (2001) developed a bi-level stochastic model to optimize the cost of a structural topology design problem. The study considered a trust topology under unilateral frictionless contact and when the load conditions data are uncertain. The scenario is one in which structural failure can only lead to reconstruction cost and not loss of life. Casas-Ramírez et al. (2018) applied the bi-level programming concept in facility location problem. The model comprises minimizing the facility cost of location and distribution by the company, which serve as the upper-level problem and maximize the facility preference by customers, which serve as the lower level problem. The cross-entropy method and the randomized greedy algorithm are used to solve the model. Wen et al. (1991) presents a comprehensive review of the BLPP applied to government policies, agriculture, economic system, financial model and transportation; however, it does not consider reliability areas. The study also analysed the geometric properties of the linear BLPP and outlined the approaches used in solving BLPP, such as vertex enumeration and its variants and the Khun-Tucker approach.
Recently, Kamal et al. (2021) studied a selective maintenance problem under a neutrosophic condition, where the authors formulated the model with fuzzy parameters. They considered a replaceable and repairable component of the system and optimized the reliability. Of all the literature on BLPP, only very few applied in reliability optimization. None of them uses the Khun-Tucker optimality conditions in maximizing the reliability of a series–parallel system. Hence, this paper aims to bridge the existing gap by demonstrating the applicability of BLPP using the Khun-Tucker approach. Therefore, the research contributes to the bank of reliability literature concerning the methodologies and techniques.
3 Methodology
This section presents an overview of the philosophy, concepts, origin and mathematical model of BLPP. It also discusses the concept of the Khun-Tucker optimality conditions as a solution approach to a BLPP. The following section discusses the BLPP.
3.1 Bi-level programming problem
The BLPP is a two-person non-zero-sum game in which the first player can influence but not control the action of the second. The BLPP is a model for a leader–follower game in which the two players try to maximize (or minimize) their objective functions. The BLPP has been developed and studied by many authors including Bialas et al. (1982), Bialas et al. (1984); Candler et al. (1982), Wen et al. (1990), Wen et al. (1991), Bard (1984) and Bard (1983). According to Nath et al. (2019), the concept is divided into two optimization levels- upper-level decision making (ULDM) and lower-level decision making (LLDM) [see Fig. 2]. At each level, decisions are taken independently; however, actions and inactions of LLDM may affect the decision-making process as a result of dissatisfaction with the ULDM outcomes. In other words, LLDM obtained its optimal solutions which satisfy its constraints as a result, create a feasible region for the ULDM who eventually make decision with its own characteristics variables with the feasible solution space created by the LLDM units.Overview of bi-level optimization.
Outline required to construct the BLPP.
-
There exist interacting decision-making units within a predominantly hierarchical structure.
-
The execution of decisions is sequential from higher to lower levels. The lower level decision maker executes its policies after and based on the decision of the higher levels.
-
Each decision-making unit optimizes its objective function independently of other units but is affected by the actions and reactions of other units.
-
The external effect on a decision-makers problem can be reflected in both his objective function and his set of feasible decisions. see Fig. 3.
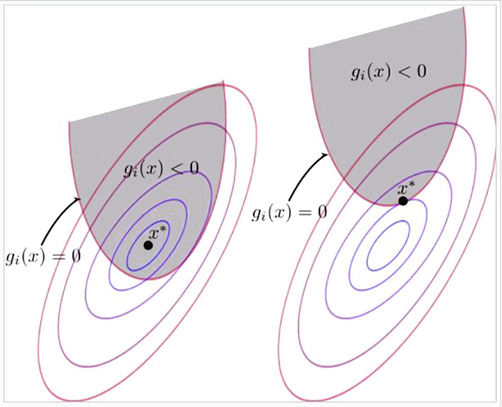
- Constraint diagram for inequality optimization problems.
Further, assume that there are two levels in a hierarchy with higher and lower level decision-makers. Let a vector of decision variables
be partitioned among the two planners. The higher-level decision maker has control over the vector
, and the lower-level decision-maker has control over the vector
. Furthermore, assuming that
are convex and bounded, the non-linear BLPP can mathematically be stated as follows:
Where
is a vector of leader’s problem variables,
vector of follower’s problem,
is a
matrix, B is a
matrix, and
and
are convex functions. Let S denotes the problem constraint region
Hence for each value of x, the lower level will react with a corresponding value of y. This induces a functional relationship between the decisions of the leader and the reactions of the follower. For a given x, let
denote the set of the optimal solution to the inner problem
,
And
represent the higher-level decision-makers solution space or the set of the rationale of the f over S, as
We assume that S is closed, bounded and non-empty with as bounded and non-empty and a unique solution exists for for any feasible x. The definitions of feasibility and optimality for the BNLPP are then given by the following:
A point is called feasible if .
A feasible point is called optimal if is unique for all and for all feasible pairs .
Thus the BLPP can mathematically be formulated as:
3.2 The Karush Kuhn Tucker (K-T) optimality conditions
An optimization problem can be linear or nonlinear in mathematical programming, and a solution can be optimal, sub-optimal, or non-optimal. For any case to happen, there are some conditions known as necessary and sufficient conditions. In a nonlinear programming problem, a solution must satisfy the first-order necessary condition alongside some regularity conditions for it to be optimal. These conditions are known as the Karush Kuhn Tucker (KKT) conditions or simply the Kuhn Tucker (KT) conditions. The conditions are named after Harold W. Kuhn and Albert W. Tucker and originated from the work published by Kuhn and Tucker (1951). These conditions later traced to the masters desertation of Karush (1939) in the study conducted by Kjeldsen (2000).
The KKT theorem in a nonlinear programming problem is a generalization of the Lagrange multipliers. The latter allows only constraints of equality nature, whereas the former takes care of the inequality constraints. The KT conditions are similar to the saddle point theorem as it spreads its global optima over the choice variables and the multipliers like Langrangean function Tabak et al. (1971).
Suppose and are differentiable continously at a point . If is a local optima and the problem satisfy the conditions given in Eqns. (6)–(10) [see Fig.], then, there exists a constant and , called the KKT multipliers, such that the following conditions hold
(a). Stationary Condition for minimization case
(6)(b). Stationary Condition for maximization case
(7)(c). Feasibility Condition for a primal case
(8)(d). Feasibility Condition for a dual case
(9)(e). Complimentary slackness condition
(10)
3.3 Stochastic Process
Some parameters, such as subsystem components, are worn out or failed in engineering systems after a successful action period. The designs are generally overhauled after some period of active action to avoid failure. However, the loss is unavoidable due to many reasons such as component lifetime, machine operators inefficiency, and overall uncertainty in the operational process. The failure rates of these parts usually follow or can be approximated to certain lifetime distributions. In the absence of data, this can be estimated using some established techniques and procedures.
Since there are several system configurations, we have different events such as the number of subsystems failures, component estimation, failure rates, and so on that can be determined concerning a particular system.
Suppose one observes random events occurring in series and assumes these events are system unit failures in time. Naturally, two central assumptions must be satisfied as follows:
-
The failures which occur disjointly in the time interval must be independent statistically.
-
The rate of these failures (average failures number per unit time) is constant and independent of any examined time interval.
For instance, any process satisfying the two conditions above is a Poisson process with a failure rate . The significant essential properties of the process are:
-
The failures number Y in an interval of time length t has a Poisson distribution having a mean t, therefore
(11) -
the times occuring between successive failures in the process is an independent random vatriables with an exponential density having a parameter , therefore
(12)Here, the MTBF is .
Several estimations of the lifetime distributions for which most reliability parameters follows has been studied and reported recently. For more details refer to Modibbo et al. (2021).
3.4 Parameter Estimation
Suppose an engineering study is to be conducted on the reliability optimization problem. Be it a system allocation problem where the objective is to allocate resources such as components in the system optimally, replace the wear-out parts, or maximize subsystem component reliability, the parameters like failure rates (MTBF, MTTF, e.t.c.) must be computed or known. If these parameters are not entirely understood, but their information or behaviour are known, then it can be estimated using statistical methods established in the literature of the subject. Most recently, Modibbo et al. (2021) proposed two estimating procedures for the reliability functions based on the Maximum Likelihood Estimators (MLEs) and Uniformly Minimum Variance Unbiased Estimators (UMVUEs). According to the authors, the system and component reliability function can be determined using formulas as follows.
The reliability function of subsystem
based on MLE can be estimated as
After obtaining the failure rates, it can be fit to observe which life distribution it follows based on that, the parameters are estimated. Two well-known approaches can be employed to ascertain the distribution suitability in such cases. They are the Akaike’s information criterion (AIC) and Bayesian information criterion (BIC) given as:
Where is the likelihood function maximized value, n is the sample size, and k is the number of parameters in the model under consideration. The model with minimum AIC and BIC values are regarded as the best-fitted distribution for the data set.
4 Bi-level Selective Maintenance Allocation Problem
Now, suppose we encounter a reliability problem of a system consisting of subsystems where it is given that a certain subsystem must be given priority over other subsystems and has control over a particular repairable component. In that case, the problem can be solved using BLPP.
Let us consider a reliability problem of a system consisting of two subsystems where the reliability of one subsystem is given priority over the other and controls specific repairable components. This way, the reliability problem can be solved by a bi-level programming problem of which two cases can be formed:
is the reliability of first subsystem.
is the reliability of second subsystem.
is the first repairable component and.
is the second repairable component.
Case 1: Here is given priority over .
is the reliability of the first subsystem that is considered as the leader’s problem where solves.
(16)Case 2: Here is given priority over .
is the reliability of the second subsystem that is considered as the leader’s problem where solves.
(17)
Procedure In the Kuhn-Tucker approach, the rational reaction set of the follower is replaced by Kuhn-Tucker optimality conditions. The leader takes into account the follower’s optimality conditions while solving its own problem. Thus, by taking the Kuhn-Tucker transformation to the inner problem, we get the resulting equivalent problem. Consider non-linear bi-level programming problem Eq. (16), and apply the K-T conditions on the inner problem subject to the same set of constarints. Here the objective is nonlinear whereas the constarints are linear. The necessary and sufficient K-T conditions for the above problem can be obtain as:
(18)Now using slack and surplus variables in the above Eq. (18), we get the KT conditions in light of Eqs. (6)–(10).
Now the non-linear BLPP is converted into a single non-linear optimization problem is:
(19)It is clear that Eq. (19) comprises of the linear equations, the non-negativity restriction, and the complementary slackness condition of KKT, while the objective function is non-linear. Now, this resulting NLPP can be solved with a variety of techniques.
5 Numerical Example
Consider a system consisting of two subsystems. The available time between two production runs for repairing and replacing back the components is 30-time units. Let the given maintenance cost of the system be 50 units. The other parameters for the various subsystems are given in Table 1. These parameters can be estimated using the process described in Section 3.3.
Subsystem
1
2
7
10
0.90
.85
5
7
8
7
3
4
To solve the above NLPP example, two cases of the problem formulated as follows:
Case 1: Here is given priority over .
is the reliability of the first subsystem that is considered as the leader’s problem where solves.
(20)
Now non-linear BLPP Eq. (20) can be solved by the procedure discussed above as follows:
Consider the lower level problem as:
Now, applying the K-T conditions in Eq. (21), we get
Now using slack and surplus variables in the above Eq. (22), we get the KT conditions in light of Eqs. (6)–(10).
Now we get the resulting single-level optimization problem as
Where I-II are the linear equations, III is the non-negativity restriction, IV is a complementary slackness condition, and the objective function is non-linear. Now, this resulting NLPP can be solved with a variety of techniques. We solve this problem with the help of a software called LINGO and get the optimal solution as .
Case 2: Here is given priority over .
is the reliability of the second subsystem that is considered as the leader’s problem where solves.
(24)
Now non-linear BLPP (24) can be solved by the procedure discussed above as follows: Consider the lower level problem as:
Now, applying the K-T conditions in Eq. (25), we get
Now using slack and surplus variables in the above Eq. (26), we get
Now we get the resulting single-level optimization problem as
Now, the resulting Eq. (27) is nonlinear and can be solved with a variety of techniques. We solve this problem with the help of a software called LINGO and get the optimal solution as .
6 Conclusion
Reliability optimization is necessary for the satisfactory performance of engineering systems. The significance of system maintenance cannot be over-emphasized. Different techniques and approaches are available for achieving the maintainability objective. This paper demonstrates the application of KKT conditions in optimizing the reliability of a system using the bi-level programming concept. The BLPP concepts have not been given much attention in the reliability study of a selective maintenance allocation problem. The KKT conditions have been derived for the BLPP in this research. Also, the necessary and sufficient conditions for the global and local optima have been obtained. The approach is then demonstrated with a numeric example under the system maintenance allocation problem. This research is novel and contributes to the bank of literature regarding bi-level optimization in reliability studies. The concept can be extended to solve using soft-computing techniques such as nature-inspired algorithms in future.
Funding
This research received no funding from any organization or agency.
CRediT authorship contribution statement
Mohammad Faisal Khan: Conceptualization, Validation, Investigation, Resources, Visualization, Funding acquisition. Umar Muhammad Modibbo: Conceptualization, Methodology, Software, Validation, Formal analysis, Investigation, Data curation, Writing - original draft, Visualization. Naeem Ahmad: Conceptualization, Validation, Resources, Visualization. Irfan Ali: Conceptualization, Methodology, Validation, Formal analysis, Investigation, Data curation, Writing - original draft, Writing - review & editing, Visualization, Supervision.
Declaration of Competing Interest
The authors declare that they have no known competing financial interests or personal relationships that could have appeared to influence the work reported in this paper.
References
- An algorithm for solving the general bilevel programming problem. Math. Operations Res.. 1983;8(2):260-272.
- [Google Scholar]
- Optimality conditions for the bilevel programming problem. Naval Res. Logistics Quarterly. 1984;31(1):13-26.
- [Google Scholar]
- A linear two-level programming problem. Computers Operations Res.. 1982;9(1):59-76.
- [Google Scholar]
- Approximating solutions to a bilevel capacitated facility location problem with customer’s patronization toward a list of preferences. Appl. Math. Comput.. 2018;319:369-386.
- [Google Scholar]
- Stochastic bilevel programming in structural optimization. Struct. Multidisciplinary Optimization. 2001;21(5):361-371.
- [Google Scholar]
- A representation and economic interpretation of a two-level programming problem. J. Operational Res. Soc.. 1981;32(9):783-792.
- [Google Scholar]
- A bi-level mathematical model for logistic management considering the evolutionary game with environmental feedbacks. The. Int. J. Logistics Manage. 2021
- [Google Scholar]
- Multi-objective bi-level supply chain network order allocation problem under fuzziness. Opsearch. 2018;55(3):721-748.
- [Google Scholar]
- Neutrosophic fuzzy goal programming approach in selective maintenance allocation of system reliability. Complex Intell. Syst.. 2021;7(2):1045-1059.
- [Google Scholar]
- Minima of functions of several variables with inequalities as side constraints. Dept. of Mathematics, Univ. of Chicago; 1939. M. Sc. Dissertation
- A contextualized historical analysis of the Kuhn-Tucker theorem in nonlinear programming: the impact of World War II. Historia Math.. 2000;27(4):331-361.
- [Google Scholar]
- Nonlinear Programming. In: Second Berkeley Symposium on Mathematical Statistics and Probability. 1951. p. :481-492.
- [Google Scholar]
- A stochastic bilevel model for facility location-protection problem with the most likely interdiction strategy. Reliab. Eng. Syst. Safety. 2021;216:108005
- [Google Scholar]
- Optimization and estimation in system reliability allocation problem. Reliab. Eng. Syst. Safety. 2021;212:107620
- [Google Scholar]
- A novel evolutionary algorithmic solution approach for bilevel reliability-redundancy allocation problem. Reliab. Eng. Syst. Safety. 2019;191:106531
- [Google Scholar]
- BLEAQ based solution for bilevel reliability-allocation problem. In: 2017 IEEE Congress on Evolutionary Computation (CEC). IEEE; 2017. p. :2661-2668.
- [Google Scholar]
- Brain Storm Optimization Algorithm in Objective Space for Reliability-Redundancy Allocation Problem. In: 2019 IEEE Congress on Evolutionary Computation (CEC). IEEE; 2019. p. :248-253.
- [Google Scholar]
- Reliability of safety-critical systems: theory and applications. JohnWiley & Sons; 2014.
- Sadati, Mir Ehsan Hesam and Nematian, Javad (2013). Two-level linear programming for fuzzy random portfolio optimization through possibility and necessity-based model. Procedia Economics and Finance 5, 657–666.
- Interactive fuzzy programming for decentralized two-level linear programming problems. Fuzzy Sets Syst.. 2002;125(3):301-315.
- [Google Scholar]
- Stackelberg solutions for fuzzy random two-level linear programming through probability maximization with possibility. Fuzzy Sets Syst.. 2012;188(1):45-57.
- [Google Scholar]
- An improved bilevel evolutionary algorithm based on quadratic approximations. In: 2014 IEEE congress on evolutionary computation (CEC). IEEE; 2014. p. :1870-1877.
- [Google Scholar]
- Optimal control by mathematical programming. SRL Publishing Company; 1971.
- Linear bi-level programming problems review. J. Operational Res. Soc.. 1991;42(2):125-133.
- [Google Scholar]
- Algorithms for solving the mixed integer two-level linear programming problem. Computers Operations Res.. 1990;17(2):133-142.
- [Google Scholar]