Translate this page into:
Bayesian estimation for parameters and reliability characteristic of the Weibull Rayleigh distribution
⁎Corresponding author. fmerovci@yahoo.com (Faton Merovci)
-
Received: ,
Accepted: ,
This article was originally published by Elsevier and was migrated to Scientific Scholar after the change of Publisher.
Peer review under responsibility of King Saud University.
Abstract
In this paper we obtain Bayes’ estimators under symmetric and asymmetric loss functions for the unknown parameters of Weibull Rayleigh distribution. When all the three parameters are unknown, the closed-form expressions of the Bayes estimators cannot be obtained. We use Lindley’s approximation to compute the Bayes estimates. The estimators have been compared through their simulated risks. We also obtain the Bayes estimators of the reliability characteristic using both symmetric as well as asymmetric loss functions and compare its performance based on a Monte Carlo simulation study. Finally, a numerical study is provided to illustrate the results.
Keywords
Reliability analysis
Maximum likelihood estimation and bayesian inferences
62F10
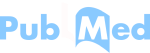
1 Introduction
In literature Weibull Rayleigh distribution has its origin in the papers by Merovci and Elbatal (2015). They discussed statistical properties of Weibull Rayleigh distribution. They proposed maximum likelihood estimation and least square estimation procedures.
The paper is organized as follows: In Section 2, we introduce the model and discussed reliability characteristic of this distribution. The maximum likelihood estimates (MLEs) of unknown parameters and reliability characteristic are obtained in Section 3. In Section 4, Bayes estimators are derived for these parameters and also for reliability characteristic. Also, using the Lindley approximation approach we have obtained Bayes estimators. In Section 5, a numerical study is performed between proposed estimates in terms of their mean square error and bias values and one data sets are analyzed for the purpose of illustration in Section 6.
2 Model
The probability density function (PDF) and cumulative distribution function of a random variable X with three parameters Weibull Rayleigh distribution are given by
We use the notation .
The Reliability function of a
distribution is of the form
In the next section we derive the maximum likelihood estimator for the parameters and and for reliability function and hazard function.
3 The maximum likelihood estimator
Consider a complete sample
taken from the model (1) the likelihood function of
and
is obtained as
Further, the likelihood equations of
and
are given by
The maximum likelihood estimates and , respectively of and , are simultaneous solutions of the Eqs. (7)–(9). The system of Eqs. (7)–(9) can not be solved analytically. So we need to apply a suitable numerical method like Newton–Raphson to obtain the desired MLEs for the given eqautions. The R statistical software is used for all the computations. In particular, the nonlinear equations are solved using nleqslv package of this software.
In the next section, Bayes estimates of unknown parameters are obtained.
4 The Bayes estimator
In this section, we obtain some Bayesian estimates of and under symmetric as well as asymmetric loss functions.The most commonly used loss function is the squared error which is symmetric in the sense that underestimation and overestimation are equally penalized. In most estimation problems the incurred losses may not be symmetric and hence use of a symmetric loss function such as squared error can not be properly justified. In practice, such situations arise in life testing and reliability estimation where incurred losses are not symmetric in general and, hence, asymmetric loss functions would be more useful to develop Bayesian procedures. Varian (1975) introduced the LINEX loss function which is asymmetric. Zellner (1986) studied further properties of this loss function and mentioned that squared error loss is a particular case of the LINEX loss function. Another useful loss function is the entropy loss function.
Recently, many authors consider Bayesian estimates for univariate distributions like: Ahmed et al. (2010), Basu and Ebrahimi (1991), Nassar and Eissa (2005) Pandey (1997), Roio (1987), Soliman et al. (2002), Soliman et al. (2005), Soliman et al. (2006), Singh et al. (2002), Singh et al. (2013b), Singh et al. (2013a) etc.
We obtain desired Bayesian estimates under stated loss functions (squared error, LINEX and entropy) which are defined as, respectively, where is an estimate of . Further, under Bayesian phenomenon, an optimal estimate relative to a given loss function can easily be obtained following the usual procedure of minimizing the average risk of with respect to a weight function usually known as the prior distribution of . For example, the Bayesian estimate, say , under the loss is the posterior mean of . Next, the Bayesian estimate of for the loss function is given by whereas under the loss function the corresponding estimate is of the form given that corresponding exist.
We derive Bayesian estimates of
, the reliability function
and the hazard function
under loss functions
and
respectively. The likelihood function of
and
is as defined in (5). Suppose that
is complete random sample taken from a
distribution. Based on this complete sample we derive corresponding Bayesian estimates of all unknowns. We assume that
and
are statistically independent and take
and
distributions as priors for these parameters. Thus, the prior distribution of
and
is of the form
Then the posterior distribution of
and
is given by
First we obtain Bayesian estimate of under the loss function using the posterior distribution . The estimate is obtained as
Next, when the loss function is we have the Bayesian estimate of as where
Finally, for the loss function we obtained that where
In a similar manner we consider deriving Bayesian estimates of and under stated loss functions.
Under the assumption that both and are unknown, expressions for Bayesian estimates of reliability function are obtained in a similar manner. In fact, under the loss function it is given as
When the loss function is we have where and finally under the loss function the Bayesian estimate of is obtained to be where
Further, the Bayesian estimate of the hazard function under the loss function is obtained as
For the loss function the corresponding Bayesian estimate is where and for the loss function the corresponding estimate is where
4.1 Lindley approximation
In previous section, we obtained Bayes estimators of
and
under various loss functions such as squared error, linex and entropy. Note that these estimators are of the form of ratio of two integrals which can not be simplified into a closed form. However, using the approach developed by Lindley (1980), one can approximate these Bayes estimators into a form containing no integrals. From practical point at view, this method provides a simplified form of Bayes estimator which is easy to apply in practice. Consider the ratio of integral I(X), where
and also, elements of the inverse of the matrix evaluated at . Other expressions are obtained as,
Now we can obtain the values of the Bayes estimates of various parameters under different loss function.(a) Case of the squared error loss function (b) Case of the Linex loss function (c) Case of the Entropy loss function
Proceeding similarly the Bayes estimators for the reliability function and hazard rate function can be evaluated under all three loss functions.
5 Numerical comparisons
In Sections 3 and 4 several estimates of and of a distribution are obtained using complete sample. The Bayesian estimates of Section 4 are obtained against different symmetric and asymmetric loss functions such as squared error, linex and general entropy. In this section performance of all these estimates have been compared numerically in terms of their mean square errors (MSEs) and average values. For our purpose we have evaluated MSEs and average values based on 5000 generations of random sample of size n from the distribution. The true value of is taken as (0.2, 0.4, 0.5) and hyperparameters are assigned values as . In each case, the Bayes estimates with respect to the loss function is computed for three distinct values of m, namely, −0.1, 0.5, 1. Also under loss associated estimates are obtained for . In addition, MSEs and average values of and are computed for two distinct values of t, namely, 2, 3.5.
In Table 51 the MSEs and average values of estimators
and
are presented for different choices of n. In each cell, all values for a particular value of n there are six rows, the first row values represent estimate of
and the second row values represent the corresponding MSE value. The third and fourth values are similarly associated with the parameter
and the fifth and sixth values are similarly associated with the parameter
. In general, we observed that Bayes estimates are superior than the respective MLEs under MSE criterion. For estimating the unknown parameters the choice
seems to be reasonable under
loss. In case of
loss, the choice
seems a reasonable choice.
n
m=-0.1
m = 0.5
m = 1.0
w=-0.5
w = 0.5
w = 1.0
20
0.243512
0.243613
0.244362
0.241534
0.23919
0.219603
0.189498
0.192441
0.044964
0.015166
0.017509
0.016438
0.014956
0.025301
0.017199
0.018149
0.473687
0.474434
0.476464
0.464162
0.453933
0.457477
0.388467
0.386258
0.070325
0.080547
0.081027
0.080182
0.077959
0.084633
0.082489
0.077869
0.602365
0.661007
0.660377
0.675875
0.665816
0.657956
0.572576
0.566286
0.206408
0.095818
0.091093
0.082383
0.075722
0.096002
0.095901
0.094711
40
0.21749
0.213914
0.214141
0.212144
0.210483
0.202679
0.191074
0.187867
0.011084
0.004917
0.005942
0.005291
0.005144
0.00414
0.003865
0.003683
0.439309
0.412514
0.412613
0.412196
0.413513
0.407034
0.405132
0.403436
0.028522
0.010853
0.011614
0.010997
0.009302
0.02961
0.025197
0.024295
0.548463
0.536169
0.538372
0.533912
0.526807
0.52273
0.527999
0.517651
0.092873
0.013446
0.024273
0.022029
0.017454
0.030178
0.026001
0.024174
60
0.211552
0.212817
0.213004
0.211375
0.209918
0.206993
0.197858
0.194267
0.005376
0.003457
0.003651
0.003567
0.002792
0.004006
0.002362
0.002305
0.427756
0.413517
0.413777
0.411974
0.410557
0.40735
0.401147
0.398316
0.017371
0.003516
0.004497
0.003903
0.003863
0.009532
0.008658
0.007815
0.524519
0.523591
0.524866
0.518613
0.51333
0.51215
0.497651
0.490168
0.047058
0.008295
0.008142
0.007366
0.007079
0.009256
0.008881
0.008801
80
0.209081
0.211491
0.211654
0.210605
0.209806
0.207755
0.200819
0.197606
0.00349
0.002209
0.002222
0.002147
0.002091
0.002043
0.001877
0.001857
0.423614
0.413096
0.413503
0.410973
0.409023
0.407593
0.398995
0.394991
0.012366
0.003346
0.003363
0.003301
0.003258
0.004573
0.004458
0.004175
0.510467
0.521683
0.522871
0.517392
0.512341
0.512888
0.494542
0.486171
0.026921
0.007576
0.007593
0.007008
0.006585
0.007293
0.006286
0.006133
100
0.206402
0.210299
0.210428
0.209651
0.209005
0.207351
0.201477
0.198742
0.002802
0.001977
0.001986
0.001933
0.001892
0.001859
0.001726
0.001702
0.415734
0.410755
0.411123
0.408934
0.407146
0.406002
0.397555
0.393695
0.009354
0.003561
0.003579
0.003480
0.003418
0.003553
0.003549
0.0034581
0.514015
0.523033
0.523891
0.519088
0.514802
0.514811
0.498813
0.490491
0.022751
0.007816
0.008110
0.008053
0.007902
0.007376
0.008246
0.006788
In Tables 52 and 53 the MSEs and average values of the estimates
and
of reliability function
are presented for different choices of t. In this case also we find that Bayes estimators show superior performance compare to the MLE. Furthermore, the squared error Bayes estimates of
has minimum MSE values among all other estimates of
. Among estimators obtained from linex loss function
gives a better choice.
n
m=-0.1
m = 0.5
m = 1.0
w=-0.5
w = 0.5
w = 1.0
20
0.791871
0.767433
0.767933
0.766425
0.766102
0.766646
0.763043
0.76292
0.00578
0.003975
0.004049
0.004465
0.004585
0.004521
0.005382
0.00555
40
0.788253
0.779303
0.779643
0.779114
0.778108
0.778708
0.776708
0.775966
0.002947
0.002108
0.002433
0.002485
0.002521
0.002291
0.002337
0.002349
60
0.785072
0.780397
0.780463
0.779957
0.779517
0.779657
0.778389
0.777841
0.001958
0.001567
0.001561
0.001570
0.001575
0.001685
0.00177
0.001763
80
0.783489
0.780571
0.78064
0.780226
0.779882
0.780032
0.779087
0.778663
0.001428
0.001170
0.001177
0.001181
0.001185
0.00121
0.001265
0.001273
100
0.78325
0.781134
0.78119
0.780855
0.780576
0.780774
0.780059
0.779703
0.001262
0.001011
0.001017
0.001021
0.001022
0.001021
0.001030
0.001035
n
m=-0.1
m = 0.5
m = 1.0
w=-0.5
w = 0.5
w = 1.0
20
0.514616
0.527684
0.530718
0.529619
0.529991
0.53047
0.528675
0.528817
0.029253
0.009876
0.013444
0.014415
0.016898
0.018317
0.026075
0.027501
40
0.512092
0.517769
0.519411
0.517908
0.517019
0.517133
0.514269
0.510783
0.006862
0.003961
0.005891
0.005915
0.006187
0.006018
0.006802
0.007134
60
0.512211
0.514399
0.514769
0.514043
0.512922
0.513329
0.509912
0.508635
0.003199
0.002557
0.002861
0.002998
0.003056
0.002699
0.002713
0.003395
80
0.513245
0.514885
0.514998
0.514296
0.513731
0.513776
0.511432
0.510449
0.002345
0.001809
0.001901
0.001903
0.001908
0.001914
0.001985
0.001991
100
0.511976
0.513229
0.51332
0.512771
0.512313
0.512326
0.510527
0.509638
0.001876
0.001561
0.001601
0.001602
0.001605
0.00161
0.001642
0.001668
The MSEs and average values of all estimates of
are presented in Tables 54 and 55. We again notice that Bayes estimates are quite superior than the MLE. The choice
with respect to the
loss produce best estimate of
.
n
m=-0.1
m = 0.5
m = 1.0
w=-0.5
w = 0.5
w = 1.0
20
0.160252
0.165234
0.164647
0.163015
0.161781
0.161103
0.164748
0.172922
0.03286
0.014645
0.010189
0.00983
0.009682
0.012783
0.012448
0.011214
40
0.15967
0.161263
0.161327
0.161002
0.160741
0.159442
0.155677
0.154505
0.001633
0.000896
0.000901
0.000896
0.000889
0.000962
0.000872
0.000901
60
0.15924
0.160761
0.16081
0.160519
0.160278
0.160036
0.156198
0.154836
0.001097
0.000670
0.000672
0.000665
0.000661
0.000688
0.000647
0.000658
80
0.158287
0.159732
0.159771
0.15954
0.159348
0.15854
0.156166
0.15501
0.000802
0.000539
0.000546
0.000535
0.000532
0.000527
0.000519
0.000522
100
0.158326
0.159593
0.159625
0.159433
0.159274
0.158547
0.156525
0.155562
0.000637
0.000460
0.000463
0.00046
0.000458
0.000459
0.000454
0.000454
n
m=-0.1
m = 0.5
m = 1.0
w=-0.5
w = 0.5
w = 1.0
20
0.536141
0.398157
0.395956
0.397877
0.3847
0.387033
0.417482
0.400376
0.123038
0.094173
0.085498
0.083405
0.07687
0.009644
0.009787
0.104902
40
0.514487
0.412248
0.412021
0.413491
0.41444
0.413399
0.422225
0.424833
0.028533
0.016722
0.016784
0.016345
0.016308
0.016363
0.016912
0.017433
60
0.506869
0.440939
0.440891
0.44092
0.441035
0.441005
0.442087
0.442777
0.009296
0.008135
0.008176
0.008033
0.007906
0.006934
0.007148
0.007268
80
0.502095
0.454709
0.454731
0.454505
0.454313
0.454415
0.453816
0.453645
0.006797
0.005074
0.005079
0.005039
0.005009
0.004896
0.004941
0.005004
100
0.499315
0.462004
0.462063
0.461715
0.461439
0.461484
0.460589
0.460214
0.004931
0.003688
0.003689
0.003683
0.003679
0.003580
0.003671
0.00367
In general mean squared error values all estimates decreases as n increases.
6 Data analysis
Two examples are presented in this section for the purpose of illustration. First, we treat a real data set.
Example 1 (real data): This data set was originally discussed by Meeker and Escobar (2014). Merovci and Elbatal (2015) fitted this data set to WR with complete sample. In this data, the times of failure and running times for a sample of devices from a eld-tracking study of a larger system. At a certain point in time, 30 units were installed in normal service conditions. Two causes of failure were observed for each unit that failed: the failure caused by an accumulation of randomly occurring damage from power-line voltage spikes during electric storms and failure caused by normal product wear. The times are: 2.75, 0.13, 1.47, 0.23, 1.81, 0.30, 0.65, 0.10, 3.00, 1.73, 1.06, 3.00, 3.00, 2.12, 3.00, 3.00, 3.00, 0.02, 2.61, 2.93, 0.88, 2.47, 0.28, 1.43, 3.00, 0.23, 3.00, 0.80, 2.45, 2.66.
The maximum likelihood estimates and different Bayesian estimates of
and
are presented in Table 6.1. The estimates of
and
are tabulated for different choices of t in Table 6.2 and 6.3 respectively. Conclusions similar to the Section 5 can easily be drawn from these tables.
m=-0.1
m = 0.5
m = 1.0
w=-0.5
w = 0.5
w = 1.0
0.27537
0.299551
0.300119
0.296669
0.293729
0.288837
0.267117
0.257319
0.292778
0.293419
0.293791
0.291564
0.289712
0.287107
0.275231
0.269985
1.56221
1.6848
1.70183
1.59505
1.50635
1.62785
1.51311
1.46127
t
m=-0.1
m = 0.5
m = 1.0
w=-0.5
w = 0.5
w = 1.0
1
0.748772
0.744989
0.745232
0.74378
0.742577
0.743375
0.740193
0.738634
3
0.115773
0.127536
0.127645
0.126985
0.126428
0.122605
0.112519
0.107982
t
m=-0.1
m = 0.5
m = 1.0
w=-0.5
w = 0.5
w = 1.0
1
0.514616
0.527684
0.530718
0.529619
0.529991
0.53047
0.528675
0.528817
3
2.96113
2.01797
2.01962
2.04233
2.09011
2.03021
2.06521
2.08488
Example 1 (simulated data): We consider a simulated data presented in Merovci and Elbatal (2015). As in previous example, here again we have obtained various estimates of
and
. These results are tabulated in Table 6.4. The corresponding estimates of
and
are presented in Table 6.5 and 6.6 respectively.
m=-0.1
m = 0.5
m = 1.0
w=-0.5
w = 0.5
w = 1.0
0.075027
0.083163
0.083227
0.082841
0.082516
0.078713
0.069954
0.066329
0.207059
0.192319
0.192957
0.18916
0.186063
0.17779
0.156861
0.150765
0.303958
0.417462
0.418344
0.412668
0.407224
0.39854
0.345298
0.316704
t
m=-0.1
m = 0.5
m = 1.0
w=-0.5
w = 0.5
w = 1.0
4
0.885334
0.880524
0.880622
0.880035
0.879548
0.879971
0.878878
0.878338
8
0.569962
0.572353
0.572639
0.570922
0.569491
0.569842
0.564855
0.562411
t
m=-0.1
m = 0.5
m = 1.0
w=-0.5
w = 0.5
w = 1.0
4
0.033615
0.030971
0.030977
0.030942
0.030913
0.030146
0.028801
0.028295
6
0.088253
0.049886
0.049833
0.050151
0.05041
0.053167
0.057698
0.059372
References
- Comparison of the bayesian and maximum likelihood estimation for weibull distribution. J. Math. Stat.. 2010;6:100-104.
- [Google Scholar]
- Bayesian approach to life testing and reliability estimation using asymmetric loss function. J. Stat. Planning Inference. 1991;29:21-31.
- [Google Scholar]
- Lindley, D.V., 1980. Approximate bayesian methods. Trabajos de estadística y de investigación operativa, 31, pp. 223–45.
- Statistical Methods for Reliability Data. John Wiley & Sons; 2014.
- Weibull rayleigh distribution: theory and applications. Appl. Math. Inf. Sci.. 2015;9:2127-2137.
- [Google Scholar]
- Bayesian estimation for the exponentiated Weibull model. Commun. Stat.-Theory Methods. 2005;33:2343-2362.
- [Google Scholar]
- Testimator of the scale parameter of the exponential distribution using linex loss function. Commun. Stat.-Theory Methods. 1997;26:2191-2202.
- [Google Scholar]
- On the admissibility of c [xbar]+ d with respect to the linex loss function. Commun. Stat.-Theory Methods. 1987;16:3745-3748.
- [Google Scholar]
- Bayesian estimation and prediction for flexible weibull model under type-ii censoring scheme. J. Prob. Stat.. 2013;2013
- [Google Scholar]
- Bayesian estimation and prediction for the generalized lindley distribution under assymetric loss function. Hacettepe J. Math. Stat. 2013
- [Google Scholar]
- Estimation of exponentiated Weibull shape parameters under linex loss function. Commun. Stat.-Simul. Comput.. 2002;31:523-537.
- [Google Scholar]
- Reliability estimation in a generalized life-model with application to the burr-xii. IEEE Trans. Reliab.. 2002;51:337-343.
- [Google Scholar]
- Estimation of parameters of life from progressively censored data using burr-xii model. IEEE Trans. Reliab.. 2005;54:34-42.
- [Google Scholar]
- Comparison of estimates using record statistics from Weibull model: Bayesian and non-bayesian approaches. Comput. Stat. Data Anal.. 2006;51:2065-2077.
- [Google Scholar]
- Varian, H.R., 1975. A bayesian approach to real estate assessment. Studies in Bayesian econometrics and statistics in honor of Leonard J. Savage, pp. 195–208.
- Bayesian estimation and prediction using asymmetric loss functions. J. Am. Stat. Assoc.. 1986;81:446-451.
- [Google Scholar]